Datacadabra's roadmap
In previous blogs, we've talked about perceiving and structuring as the first 2 of the total 4 steps that make up Datacadabra's AI roadmap. In this blog, we explain how you can learn to understand and analyze the information you get from data. We do this using a model developed specifically for this purpose. We'll come back to that later in this blog.
Recognizing patterns
In step 3 of our roadmap, we are to the point where we can train the model based on a constructed labeled dataset. That is, we can have the model recognize things based on certain observations. For example, whether it is a picture of a dog or a cat. The training then consists of the input of a large amount of pictures depicting dogs or cats. Based on the input, the model learns to recognize certain patterns.
By continuing to train a model, you can make the model detect objects more accurately and perform better. Does the outcome, or is the percentage of positive observations sufficient? Then we can implement the model in a production environment. If not, we add new data to the dataset, start training and evaluating again. Just until we have a suitable output.
Learning to better understand input data
In our previous blog, we told about the Digital Intelligence Framework (DIF), a blockchain of technologies and tools that enables us to effectively develop, implement and manage digital intelligence, such as artificial intelligence (AI) and machine learning (ML). Using those tools and technologies, we come up with a model that allows us to better analyze and understand the input data. But also a model that, when it meets expectations, can be reused to save costs with it
Three models
When choosing which model to work with, computing power, accuracy, speed and cost are the most important factors to weigh against each other. There are 3 main types of models within computer vision that you can train with:
Classification Model
Classification models are used to make decisions or assign items to categories. For example, a classification model produces so-called boolean output (true or false) or categorical decisions, such as "cat" or "dog."
Object detection model
This type of model is usually trained to detect the presence of specific objects, for example, a dog or a cat.
Segmentation Model
Which pixel belongs to which category? So, which pixels make up a cat or a dog? A segmentation model is highly accurate and requires more computation time. The more demands you place on the computational power, accuracy and speed of the model, the more impact that ultimately has on the final cost of the model to be developed.
Which pixel belongs to which category? So, which pixels make up a cat or a dog? A segmentation model is highly accurate and requires more computation time. The more demands you place on the computational power, accuracy and speed of the model, the more impact that ultimately has on the final cost of the model to be developed.
The advantage of the models mentioned above is that they can all be applied in images, videos or real-time operations. In particular, the models we develop are aimed at helping humans and AI work better together in labor-intensive environments. Applying smart AI technology here enables faster, better and more cost-efficient production. Without sacrificing jobs. The application possibilities of AI in an originally labor-intensive process are inexhaustible.
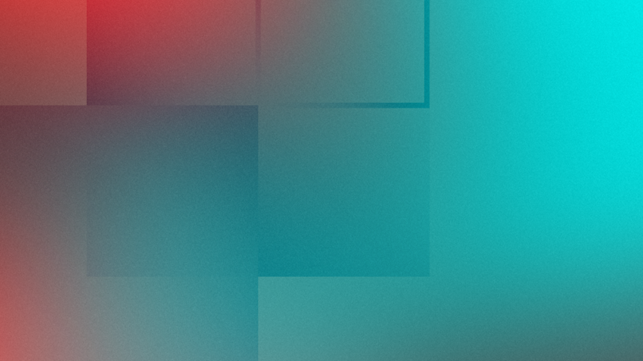
Wondering if AI also fits your business environment? And would you like to read more about our unique roadmap? We previously published a blog on our website about the first two steps. And soon we will share with you the fourth and final step, about implementing models in a production environment.
The white paper DIF in your mailbox?
Datacadabra has created a white paper in which we explain how the DIF works. Using an example, we take you step-by-step through the process of structuring and understanding data perception so that models can be trained on it.
Curious about the whitepaper? Fill in your details below and you will receive our white paper on the DIF in your email